Projects
A Project
corresponds to an automated Cleanlab analysis of your Dataset as well as corrections to improve the Dataset.
Once your dataset is successfully ingested, it will populate in the “Datasets” section. The next step is to select Create Project
under the Action column.
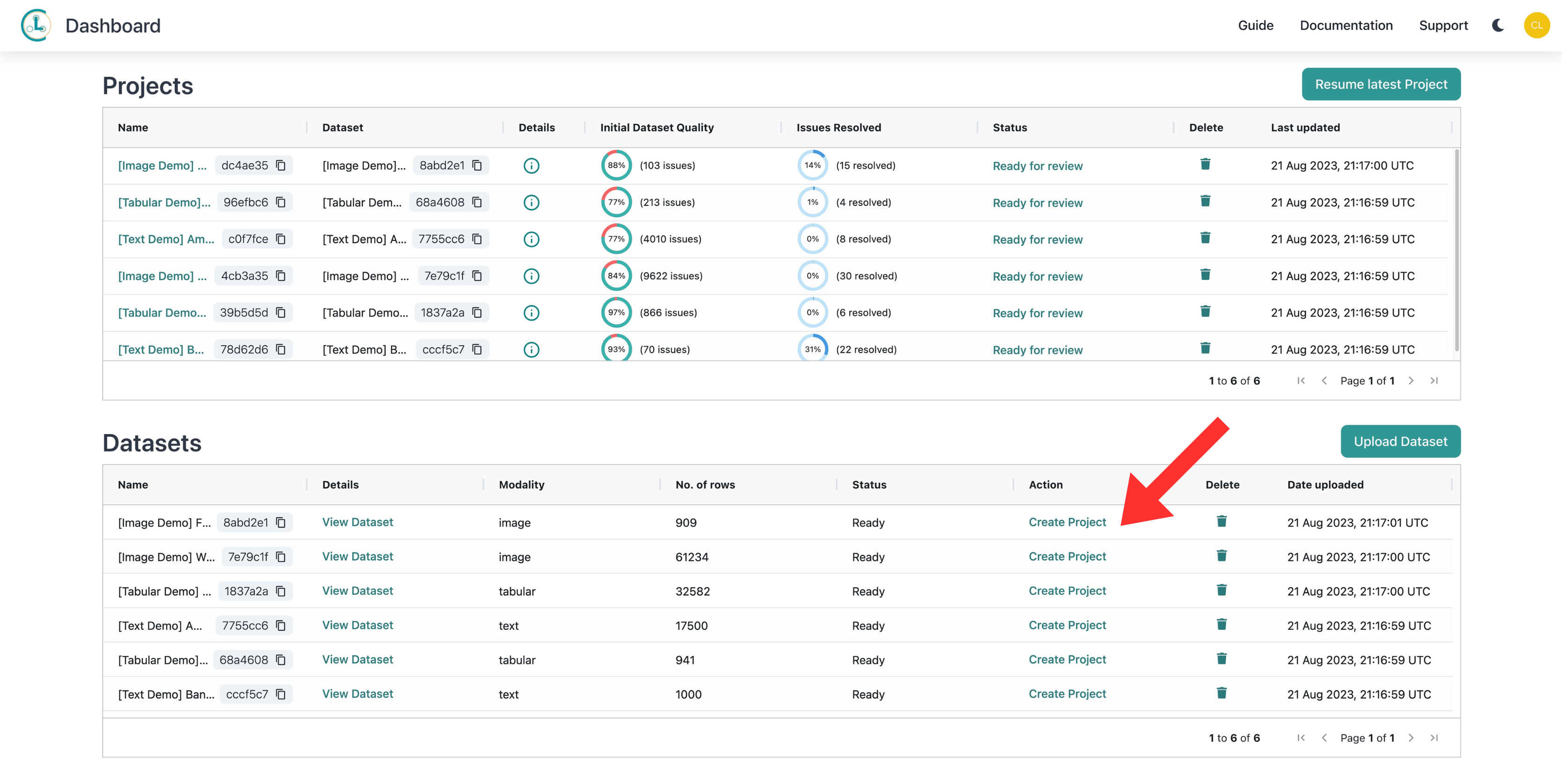
As soon as you create a Project, Cleanlab Studio automatically trains ML models to analyze your data. This may take some time (you will get an email when the results are ready). Once the Project Status shows “Ready for review”, you can click the Project to review the issues in your dataset and start correcting them.
Creating a project to improve your data requires few selections that are explained below. Refer to our tutorials for specific examples. If you are unsure about anything, just ask: support@cleanlab.ai
Machine Learning Task / Dataset Type
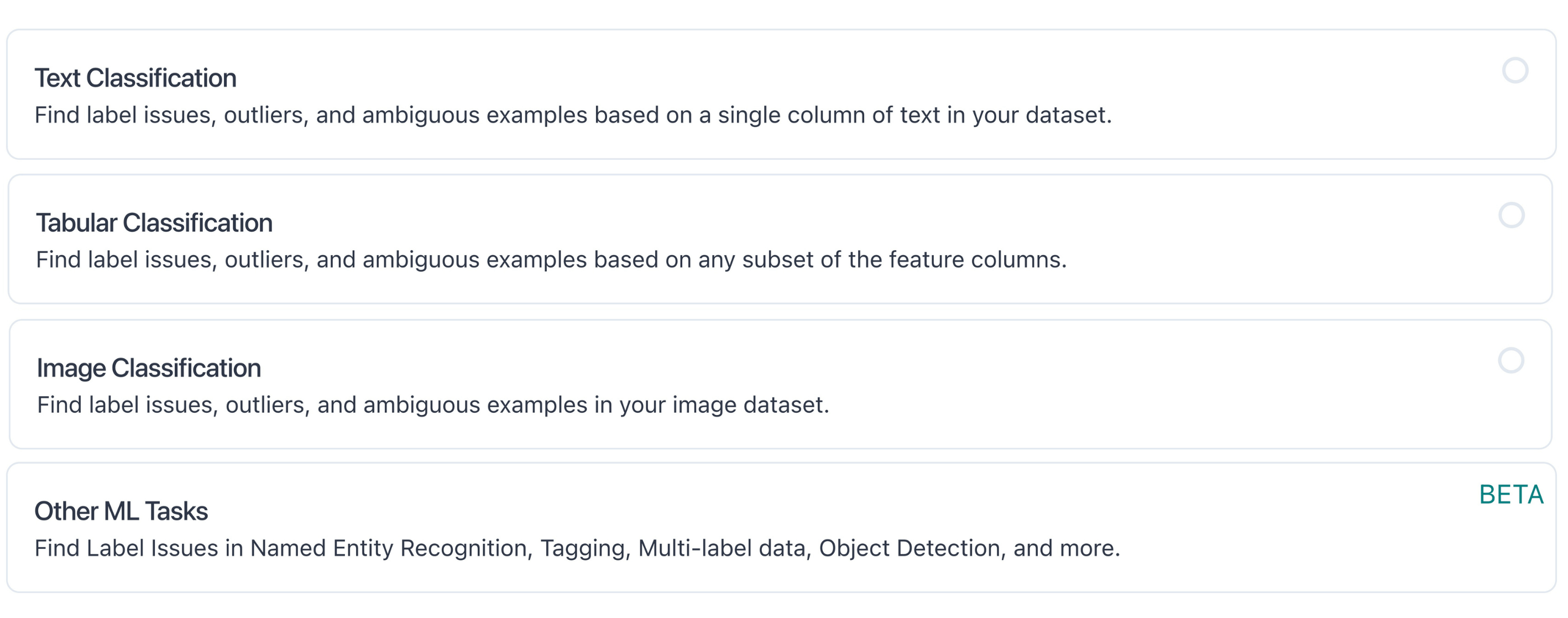
This selection corresponds to the modality of your data. Cleanlab Studio supports the following data modalities:
- Text — create a project to analyze a single column of text data (e.g. customer service requests)
- Tabular — create a project to analyze multiple columns of data (e.g. financial reports)
- Image — create a project to analyze image data (e.g. e-commerce products)
Each Project uses AI to analyze your data, which involves training certain types of ML models appropriate for your dataset and the specific information you are interested in. Cleanlab Studio supports the following ML tasks:
- Multi-class classification (
"multi-class"
) : A single example belongs to exactly one of the classes – the classes are mutually exclusive. - Multi-label classification (
"multi-label"
) : A single example can belong to one or more classes simultaneously or none of the classes at all – the classes are not mutually exclusive (each class either applies to the example or not).

Additionally, the following ML tasks are supported through Cleanlab Studio’s Python API only:
- Regression (
"regression"
) : Each data point has a target variable with continuous/numeric values (i.e. price, income, age) that we’d like to predict/impute or detect errors in. Currently only supported for text and tabular datasets. - Unsupervised/No Task (
None
or"unsupervised"
) : There are no labels associated with data points (note: this is not the same as an unlabeled dataset you wish to get labeled). Currently only supported for text and image datasets.
The above enumerates what is currently supported in the generally available version of Cleanlab Studio. Other types of datasets and machine learning tasks are supported in private Enterprise plans (documents, audio, video, image segmentation, object detection, …).
Help: Don’t fret if you are not a Machine Learning expert! Which ML task to choose simply depends on what information in the dataset (e.g. which column of a table) you would like to predict/impute values of or detect erroneous values in. We call entries in this column the label for each data point (row).
- If there is no specific label column/variable of interest in your dataset, select “unsupervised” as the ML task type.
- If the label for your dataset is numeric values (e.g. price, income, age), select “regression” as the ML task type.
- If the label for your dataset is categorical (e.g. yes/no binary variable, or one of say 7 possible values for each data point), select: “multi-class” (classification) as the task type.
- If the label for your dataset is a list of multiple categories (e.g. image/document tagging where there are say 7 possible classes and each data point belongs to some subset of the classes simultaneously), select: “multi-class” (classification) as the task type.
- If the label for your dataset is arbitrary open-ended text (e.g. LLM instruction-tuning datasets and other sequence-to-sequence tasks with input+output text pairs), you should instead use Cleanlab’s Trustworthy Language Model.
We emphasize the choice of ML task is just about choosing what piece of information in your data you are specifically interested in analyzing more closely (i.e. what form of information your chosen label corresponds to). For structured/tabular datasets, you have the option to select which columns Cleanlab will use as predictors of this label when training ML models.
Cleanlab Studio is so easy to use, even non-ML experts can apply highly-accurate Machine Learning. Using the Model Deployment
feature of a Project, you can use Cleanlab’s ML model to predict the value of labels for new data points (via a drag-n-drop interface in the Web Application, or in real-time via a REST API and our Python API).
Model Type
Cleanlab trains many different ML models on your data and automatically identifies the best in order to detect data issues in your dataset. This process can take some time to deliver the best results. You have a choice between two settings:
- Fast — trains faster but less accurate ML models
- Regular — trains more accurate ML models and runs more data analyses
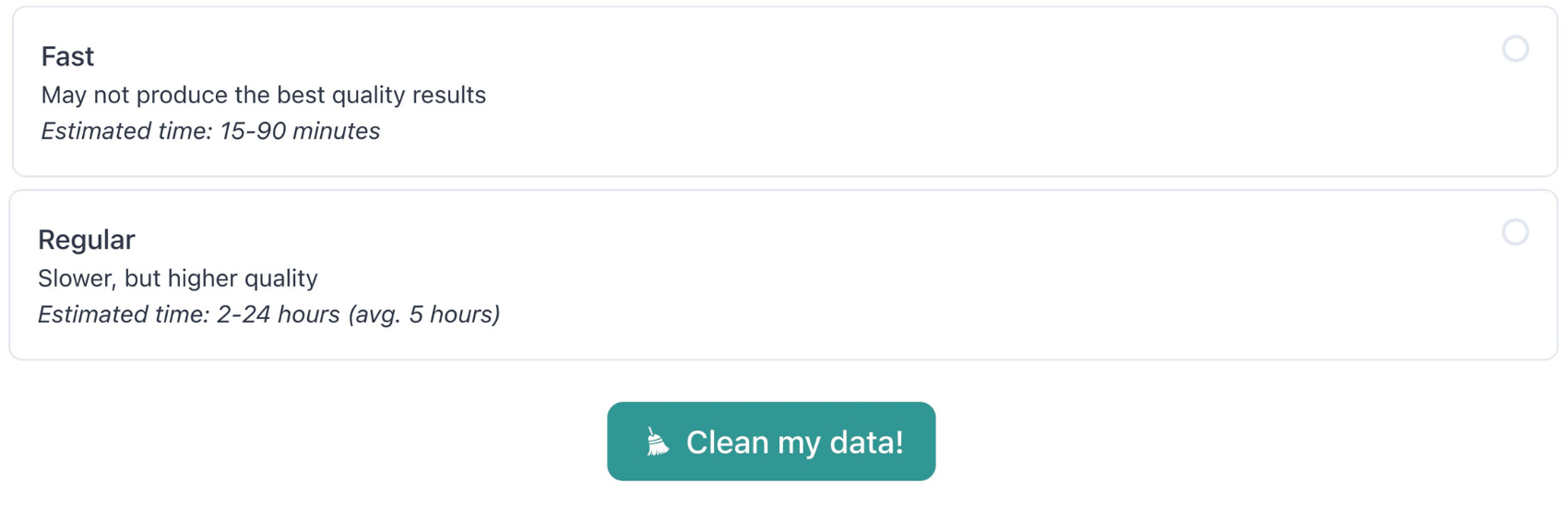
We recommend using Regular mode, except when you have a tight deadline to meet.
After making your selections, click Clean my data!
to kick-off project training.
You will get an email when training completes.
At that point, the auto-detected data issues are presented in an intuitive
data correction interface for you to quickly clean up your dataset.
Custom model training time-limits for bigger datasets are supported in private Enterprise plans.